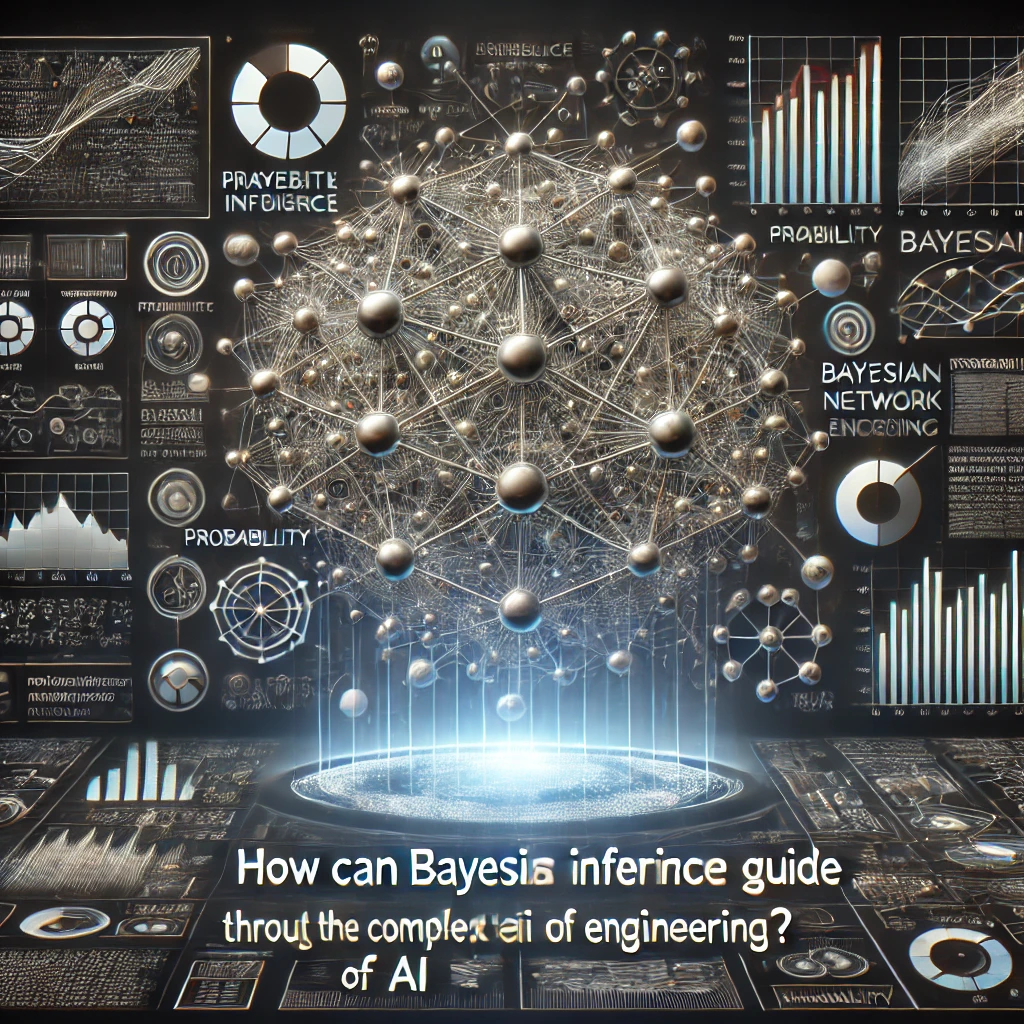
Most AI engineers navigate through a labyrinth of uncertainties, probabilities, and complexities daily. In this guide, we will explore how Bayesian inference acts as a steadfast beacon, illuminating the path to effective decision-making in AI engineering. By harnessing the power of Bayesian statistics, engineers can make sense of data ambiguity, tailor machine learning models for optimal performance, and mitigate risks associated with erroneous predictions. Let Bayesian inference be your guiding light in the intricate world of AI engineering.
Key Takeaways:
- Bayesian inference is a powerful tool that can help in making decisions in AI engineering by incorporating prior knowledge and updating beliefs based on new evidence.
- By using Bayesian inference, engineers can make informed choices about model selection, hyperparameter tuning, and uncertainty quantification, which are critical aspects of developing robust AI systems.
- Bayesian inference provides a principled framework for reasoning under uncertainty and can guide AI engineers through the complex terrain of navigating trade-offs between model complexity, performance, and interpretability.
Understanding Bayesian Inference
Basic Principles of Bayesian Statistics
On understands Bayesian statistics as a framework for reasoning and decision-making under uncertainty. It is based on the idea of using probability to represent uncertainty about the unknown quantities in a given problem. The basic principles involve updating beliefs based on new evidence, leveraging prior knowledge, and making predictions by incorporating uncertainty.
Types of Bayesian Models in AI
Now, Bayesian models in artificial intelligence (AI) can be broadly categorized into two types: Generative models and Discriminative models. Generative models learn the joint probability distribution of the input features and the target labels, while Discriminative models directly model the conditional probability of the target labels given the input features. The choice between these models depends on the specific problem at hand and the available data.
Generative Models | Discriminative Models |
---|---|
Learn joint probability distribution | Model conditional probability |
Can be used for data generation | Focus on decision boundary |
More complex and computationally intensive | Simpler and faster training |
Require additional assumptions | Directly predict target labels |
Can handle missing data well | Less sensitive to noise |
Step-by-Step Application of Bayesian Inference in AI
Even in the vast landscape of AI engineering, Bayesian inference provides a structured approach to deriving insights from data, making it an invaluable tool for decision-making and model development.
Setting Up the Bayesian Framework
The | Description |
Data Collection | Collect relevant data points to form the basis of your Bayesian model. |
Model Selection | Choose the appropriate Bayesian model that best fits your data and problem statement. |
Tips for Effective Implementation
Key | Points |
Data Preprocessing | Ensure data cleanliness and consistency before applying Bayesian inference techniques. |
Prior Selection | Choose informed priors that reflect existing knowledge about the problem domain. |
Model Evaluation | Use techniques like cross-validation to assess the performance of your Bayesian model. |
Effective implementation of Bayesian inference in AI involves careful consideration of data quality and prior knowledge incorporation. Bayesian models can provide valuable insights when implemented correctly, but overlooking key steps in the process can lead to biased results or inaccurate predictions. Utilizing appropriate data preprocessing techniques, selecting informative priors, and rigorously evaluating model performance are crucial for successful Bayesian inference in AI. Any oversight in these areas can compromise the integrity and reliability of your AI system.
Factors to Consider in Bayesian AI Engineering
Once again, when delving into Bayesian AI engineering, there are several crucial factors to consider that can guide you through the complex terrain of this field. It is crucial to take into account data quality, the complexity of the model, the computational resources available, the interpretability of results, and the uncertainty quantification in your analysis. Knowing how these factors interplay can help you make informed decisions throughout your engineering process.
Pros and Cons of Bayesian Methods
Pros | Cons |
Account for uncertainty | Computational intensity |
Regularization of parameters | Interpretability challenges |
Effective on small data sets | Subjective choice of priors |
Can incorporate domain knowledge | Sensitivity to model specification |
Selecting the Right Tools and Techniques
Factors such as the nature of your data, the complexity of your problem, and your computational resources must be considered when selecting the right tools and techniques for Bayesian AI engineering. If you choose the right tools and techniques, you can improve the efficiency and effectiveness of your models, leading to more accurate results. It is crucial to stay informed about the latest developments in the field to ensure you are utilizing the best tools for your specific project requirements.
Real-World Applications and Outcomes
Enhancing Decision-Making with Bayesian Inference
Now, let’s explore how Bayesian inference can enhance decision-making in AI engineering. By incorporating probabilistic reasoning into the decision-making process, Bayesian inference enables engineers to make more informed and reliable decisions. This approach considers uncertainty and updates beliefs as new data is received, leading to more accurate and robust decision-making outcomes.
Improving Predictive Quality in AI Systems
With Bayesian inference, AI systems can significantly improve predictive quality by taking into account uncertainty and incorporating prior knowledge. By leveraging Bayesian techniques, AI engineers can strengthen the predictive power of models, leading to more reliable and trustworthy results. This can drastically reduce the risk of making critical errors and enhance the overall performance of AI systems.
Summing up
With these considerations in mind, it is evident that Bayesian inference can be a powerful tool for navigating the intricate landscape of AI engineering. By providing a framework for incorporating uncertainty, prior knowledge, and updating beliefs based on new evidence, Bayesian methods enable engineers to make more informed decisions and optimize their models effectively. This approach not only enhances the interpretability and reliability of AI systems but also fosters a more robust understanding of the underlying data generating processes. Embracing Bayesian inference in AI engineering can lead to more efficient model training, better risk assessment, and ultimately, more impactful and reliable artificial intelligence applications.
FAQ
Q: What is Bayesian inference?
A: Bayesian inference is a method of statistical inference in which Bayes’ theorem is used to update the probability for a hypothesis as more evidence or information becomes available.
Q: How can Bayesian inference guide you through the complex terrain of AI engineering?
A: Bayesian inference can guide you through the complex terrain of AI engineering by providing a principled framework for dealing with uncertainty, incorporating prior knowledge, updating beliefs based on data, and making optimal decisions under uncertainty.
What are the benefits of using Bayesian inference in AI engineering?
A: The benefits of using Bayesian inference in AI engineering include the ability to: handle uncertainty in data and models, incorporate prior knowledge in a principled way, quantify uncertainty in predictions, make data-efficient decisions, and adapt to new data in a flexible manner.